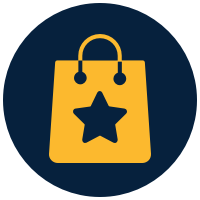
Overview
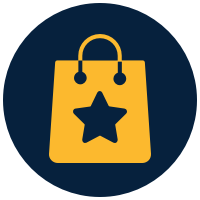
Syllabus
Intro
Discriminative vs. Generative Models • Discriminative model: calculate the probability of output given
Quiz: What Types of Variables?
Why Latent Random Variables?
An Example (Goersch 2016)
Problem: Straightforward Sampling is Inefficient
Solution: "Inference Model" • Predict which latent point produced the data point using inference
Disconnect Between Samples and Objective
VAE Objective • We can create an optimizable objective matching our problem, starting with KL divergence
Interpreting the VAE Objective
Problem! Sampling Breaks Backprop
Solution: Re-parameterization Trick
Generating from Language Models
Motivation for Latent Variables . Allows for a consistent latent space of sentences?
Difficulties in Training
KL Divergence Annealing
Weaken the Decoder
Discrete Latent Variables?
Method 1: Enumeration
Reparameterization (Maddison et al. 2017, Jang et al. 2017)
Gumbel-Softmax • A way to soften the decision and allow for continuous gradients
Variational Models of Language Processing (Miao et al. 2016)
Controllable Text Generation (Hu et al. 2017)
Symbol Sequence Latent Variables (Miao and Blunsom 2016)
Taught by
Graham Neubig